#103- Reinventing drug discovery
Transforming a billion-dollar, decade-long gamble into a faster, cheaper, and smarter process with higher odds of success.
Drug discovery is very much like venture capital. Long, arduous, expensive game which has high stakes, low odds of success but has blockbuster outcomes upon success. Common discourse is that it takes more than a billion dollars and a decade to get a drug from research to pharmacy shelves a long, expensive game with high stakes and low odds.
Researchers go through a series of stages:
Target identification - finding a biological target for the drug
Hit discovery - testing thousands of compounds to hit that target
Lead optimization - improving promising compounds for better efficacy and fewer side effects, and
Preclinical testing - lab and animal tests for safety
Clinical testing - testing on humans from phase 1 - 3
Despite this, less than 1 in 10,000 compounds end up on pharmacy shelves. Failures happen when a drug doesn’t work as hoped, causes unexpected side effects, or does not trigger the desired response.
This high failure rate, combined with the sky-high development costs (some even estimate as high as $2.6 billion1 per successful drug, counting all the failed attempts), makes drug development one of the riskiest, most capital-intensive fields around. Companies end up prioritizing safe bets—common diseases and chronic conditions—rather than investing in groundbreaking but riskier therapies.
But what’s wrong with the traditional way of discovery?
Drug discovery has been around for decades, but the process hasn’t aged well. It’s slow, expensive, and riddled with inefficiencies. While traditional methods like high-throughput screening, pre-clinical testing, and clinical trials have delivered life-saving drugs, they’re now struggling to keep up with demands for faster, cheaper, and more precise therapies.
High-Throughput Screening (HTS) gives early hits, but with low return
HTS is where it all begins. Massive libraries of chemical compounds are tested in labs to find promising "hits." But it’s a grind:
Expensive and resource-heavy- Testing thousands to millions of compounds racks up costs for synthesis, equipment, and manpower
Low success rates- Most “hits” go nowhere, failing to deliver results in later stages
Simplistic models- Testing in 2D cell cultures or isolated systems often misses the complexity of real biology, leading to high failure rates
HTS struggles because it’s built for scale, not precision. Screening millions of compounds means casting a wide net, but most “hits” aren’t viable due to a lack of biological depth. The 2D cell cultures and isolated assays used in HTS oversimplify real-life biology, missing key factors like tissue interactions or metabolic effects. This disconnect explains why compounds that look good in a lab often fail in advanced testing. The process trades accuracy for volume, leading to high costs and low payoff. The core issue with HTS is that biology isn’t simple. Living systems are dynamic, interconnected, and influenced by countless variables. HTS, however, relies on simplified models—static 2D cell cultures or isolated assays—that don’t replicate these complexities. As a result, compounds that seem promising in the lab often fail in real-world scenarios. Add to that the sheer scale of testing and the lack of predictive precision, and you’re left with a process that’s inherently inefficient. It identifies "hits," but most aren’t biologically meaningful when tested further.
Pre clinical testing is slow, costly, and often inaccurate
After HTS, potential drugs face animal testing to assess safety and efficacy. This step, while necessary, has its flaws:
Time-consuming- Pre-clinical studies take years, delaying progress to human trials
High costs- Animal studies and skilled personnel aren’t cheap
Ethical issues- Animal testing faces growing pushback, and results often don’t translate well to humans, leading to wasted effort
The inefficiencies of pre-clinical testing come down to the limitations of animal models and the complexity of biology. Animals don’t replicate human systems perfectly, so drugs that seem safe or effective in animals often fail in humans. Testing a drug’s behavior (toxicity, metabolism, etc.) involves many detailed experiments, which are time-intensive and costly. Ethical concerns add pressure to reduce animal use, but alternatives aren’t yet reliable enough to replace them. This combination of biological mismatch, resource demands, and ethical scrutiny makes pre-clinical testing a slow and uncertain process.
Trial progression morphs into a high-stakes gamble
Clinical trials require the most amount of money and time are spent
Despite the time investment, the odds of success are slim because a very high percentage of drugs in clinical trials fail, mostly in Phase 2 or 3 due to efficacy issues.
This means getting a drug to the market is expensive and lengthy. Discovery & trials can run for years, costing millions to billions of dollars per drug. Every failed drug racks of an enormous amount of failed R&D spends. Having enough failed drugs means companies move towards molecules and diseases which are safer and easier to cure - making the healthcare gap even wider despite billions invested.
The big picture is that this is a model under extreme pressure. The foundational traditional drug discovery process is fast becoming unsustainable. Time and costs are prohibitive. Most investments fail with late-stage failures causing maximum pain. Patient specific outcomes are eventually ignored leading to less effective therapies and we’re not even mentioning the ethical issues regarding animal testing.
AI and insilico technologies (i.e. computer aided) are changing this. AI is not only reducing developmental costs but also reducing developmental times. And as we can leverage ML on massive amounts of data to uncover the inefficiencies of the current, traditional process, maybe we can also improve success rates by moving towards a more a predictive, data-driven, and personalized future for drug development.
AI & Insilico- The next frontier in drug discovery?
AI and insilico methods are shaking up drug discovery. The days of spending a decade and billions of dollars hoping for a breakthrough seem to be slowly but surely in the past. Now AI-driven models and computer simulations are speeding up the process, reducing costs, and making predictions that are far more accurate. How?
Faster Discovery
Traditional drug discovery takes 10–15 years to identify viable compounds. AI and insilico models can cut that timeline down by analyzing massive datasets—from genomics to chemical libraries—faster than any lab. Rather than testing thousands of compounds in the lab, AI finds the top candidates based on pattern recognition. Companies like Atomwise and Exscientia are already using AI to design molecules, predicting their effectiveness before anything hits the lab bench. Insilico methods go even further, simulating how molecules interact with targets in virtual environments. This cuts both the time and cost of finding a lead compound since fewer resources are spent on trial and error in the lab.
More Precision
Traditional methods often miss the mark because biological systems are complex and hard to predict. Insilico models, however, use simulations to predict how drugs will behave in real-world biological systems, allowing researchers to pinpoint targets more accurately. For instance, DeepMind’s AlphaFold has cracked the protein-folding puzzle, helping to identify precise drug targets and boosting success rates in clinical trials.
AI also improves toxicology prediction by simulating interactions across organs and systems, flagging potential side effects earlier. This enables safer drugs and more efficient compound optimization.
Cost Savings
Traditional drug development is prohibitively expensive, with costs averaging $2.6 billion when factoring in failed attempts. Insilico methods and AI dramatically lower these expenses by cutting down on the need for large-scale compound production, long preclinical trials, and lengthy regulatory steps.
AI and insilico methods are here to stay, not as enhancements but as essential tools driving the next wave of breakthroughs in drug discovery.
Why AI is primed to make drug discovery better, faster, cheaper?
Better computing power creates level playing field
Advancements of GPUs, TPUs, and quantum computing have dramatically reduced the time (and maybe cost, still on the fence here) of running complex simulations. Tasks which once took months are now done in merely hours. This rapidly reducing timeline has made decoding biological complexity relatively democratic, enabling AI to crunch massive datasets and make predictions that were previously out of reach. Maybe in the future, quantum computing and supercomputers can promise even finer simulations of molecular interactions to enable smarter drug design.
Bring data back on the forefront
We have definitely entered an era of data - its no longer a bottleneck, instead abundant data is proving to be a massive opportunity.
Genomics- Due to cheaper sequencing at scale due to AI, we now have the ability to find disease targets and predict patient responses.
Molecular interactions- Studying accurate molecular interactions beyond genetic and metabolic and proteomic data becomes a reality
Trial data- AI can sift through trial data to nudge discovery teams to go after higher probability of success molecules
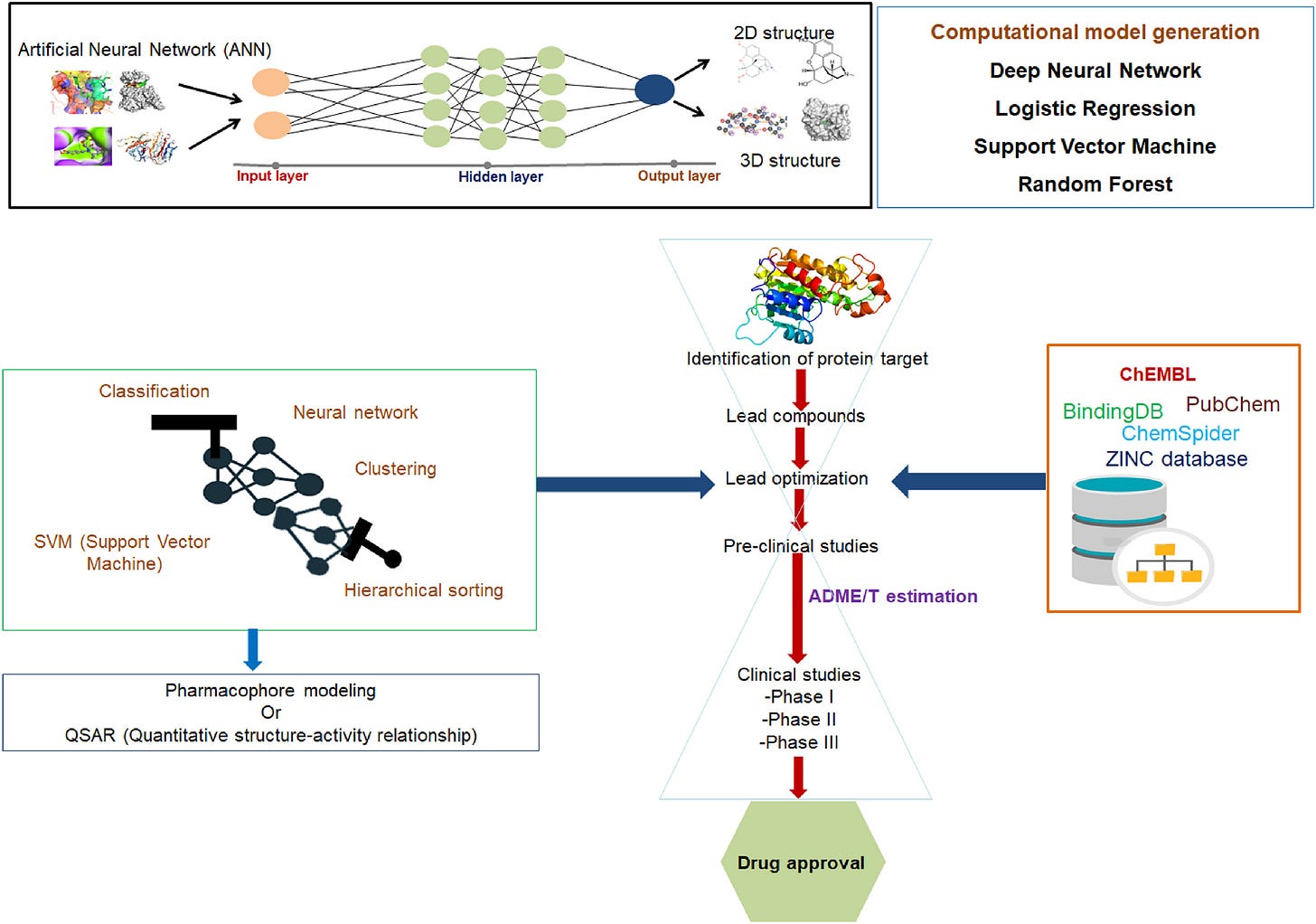
Smarter ‘everything’
AI breakthroughs like deep learning, generative models, and reinforcement learning have changed the game:
Deep Learning- As seen earlier, deep learning models like AlphaFold are solving decades-old problems like protein folding.
Generative Models- AI can now design new molecules, automating a process that was once manual and slow.
Reinforcement learnings- Like muscle memory, AI can learn from repeated iteration to improve (and maybe eventually perfect?) drug design.
NLP- AI sifts through a ton of scientific literature, pulling insights at an unparalleled speed and accuracy compared to humans
Enabling cross-ecosystem partnerships
The AI & pharma ecosystem is built on the back of partnerships. Tech startups and pharma giants are coming together to pool resources. This means big pharma can tap into AIs speed and innovation while reducing its investment time, energy & risk of R&D. At the same time collaborations and pharma backing can mean rapid validation of business and technological sanity check for tech startups. This means no promising lead is left on the table.
Faster, smarter drugs for a demanding and deserving market
The pandemic proved drug timelines can shrink when the pressure’s on. But so much is still left on the table. Demand is surging for treatments tailored to patients, for better quality of life, and backed by data. AI promises to deliver faster timelines, better outcomes, and more personalized solutions, making it the catalyst pharma needs to rethink its playbook.
In my next post, I will discuss some of the specifics of AI drug discovery, consolidation in the space, why everything is not as rosy as it seems, and where I see pockets of opportunity.